Classification and Feature Selection of Cancer Tumors Using Advanced Machine Learning Techniques

​
Cancer is an incurable disease that affects people in numerous types, the most common in the United States being breast, lung, prostate, and colon cancer. A study predicted that in 2020, around 1.8 million people will have been diagnosed with cancer in the US alone; 606,520 of those patients have been estimated to die from the disease. Being there is no cure yet, treatments such as surgery, chemotherapy, and radiation are available in addition to cancer immunotherapy, a form of therapy aiming to fight cancer through either activating or depressing the immune system. In the past, machine learning has been used to acquire a more accurate prognosis of the disease by developing a model of treatments of certain cancers, as well as identifying useful elements of datasets. Current research through machine learning models are being utilized to make cancer immunotherapy more accurate in providing an understanding of the genotype-phenotype relationship of cancer tumors and whether or not computational tools can be sufficient in classifying such tumors. The classification of cancer tumors is an open problem, and an effective tool for doing so has the potential to significantly better patient outcomes through a more accurate and quick diagnosis process. In this paper, machine learning techniques are used to identify which genes are most significant in giving rise to distinguished types of cancer tumors. Four different machine learning methods were tested to predict whether or not gene expression values could accurately predict cancer tumors, effective in elucidating that there is a strong relationship between genes and the type of tumor. Then, Random Forest feature selection was used to calculate the 50 and then 25 most significant genes in giving rise to a certain type of cancer. Then, the four models were trained/tested again on the feature selected dataset to determine if predictive capability was maintained. We discovered that a highly accurate machine learning classifier can be created a small number of significant genes instead of a whole genome.
Meet The Team
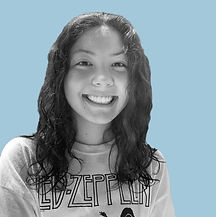
Bianca Jortner
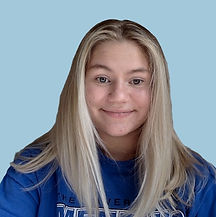
Madeleine Mejia
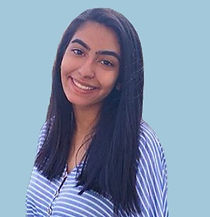