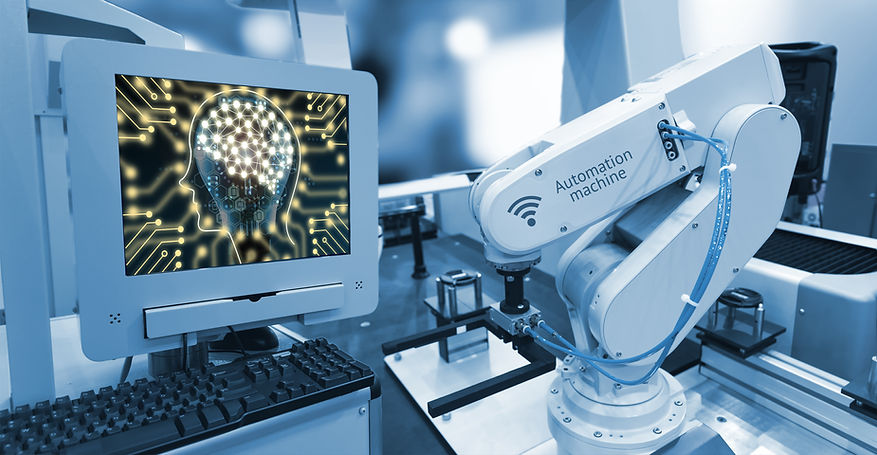
​
Autism is a developmental disorder that is often identified by complications with social interaction and communication. Even though knowledge about it has increased and instruments such as the ADOS and ADI are available to assess the disorder, it is usually distinguished from observable symptoms, which makes it especially difficult to diagnose. A variety of concerns arise as a result of this issue, one of them being the costs, which can be reduced with early screening. This research focuses on improving the diagnosis pipeline of autism by training an Autism Spectrum Disorder dataset from the University of California, Irvine with machine learning models. We first designed a neural network and Random Forests model, and trained/tested them to identify the presence of autism in toddlers. We also used feature selection through Random Forests to identify which clinical characters are most significant in giving rise to autism. The outcome of this study was a highly accurate classifier for predicting the presence of autism and significant information on the importance of several physical characteristics in indicating autism. This enhanced diagnosis is critical as it leads to a more personalized and early-stage treatment, which can alleviate the effects of autism thereafter.
​
Awards & Publications:
-
IEOM 2021 Singapore 3rd in Applied Projects
-
Indexed & Published in Elsevier
Using Machine Learning to Predict Autism in Toddlers
Meet The Team
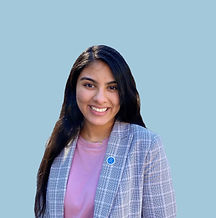
Zoya Farooqui
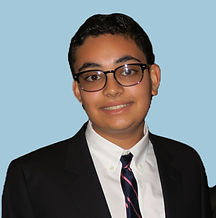
Branden Sattler
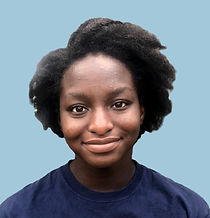
Unyime Usua
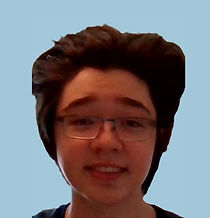_edited.jpg)